Parameters¶
Many plugin components, including datasets, recipes, macros, and webapps, can be configured through a form.
The list of parameters required by a given component must be specified as a JSON array in the params
field of
the JSON configuration file of the component and DSS will generate the corresponding user interface.
Describing parameters¶
Each parameter is a JSON object with the following fields:
type
: Type of the parameter. The most common types are STRING, INT, DOUBLE, BOOLEAN, SELECT, DATASET and COLUMN. This field is mandatory.name
: Name of the parameter as accessible by your component’s code, in the configurationdict
. This must be a valid variable name in Python and Javascript. We highly recommend that you_use_slug_like_names. This field is mandatory (except for the type SEPARATOR, see below).label
: The user-visible name that appears in the form. This field is mandatory.description
: Additional help to describe the parameter. Will appear on the side of the form.defaultValue
: The pre-filled value of the parameter. The type must match the field type.mandatory
: true/false. When true, this parameter must be supplied to use the component. When false, the parameter is optional.visibilityCondition
: Show/hide this parameter depending on a condition. See Conditional parameters for details.
Besides, additional fields may be available for specific types of parameters. See below.
To get the value of a parameter, in Python, we recommend using the form
config.get(param_name, default_value)
to give a default value to each parameter or use the field defaultValue
.
Available parameter types¶
There are many different types of parameters, but they can be grouped into:
Basic types: BOOLEAN, DATE, DOUBLE, DOUBLES, INT, PASSWORD, STRING, STRINGS, TEXTAREA.
Complex types: ARRAY, KEY_VALUE_LIST, MAP, MULTISELECT, OBJECT_LIST, SELECT.
DSS object parameters: API_SERVICE, API_SERVICE_VERSION, BUNDLE, CLUSTER, CODE_ENV, COLUMN, COLUMNS, CONNECTION, CONNECTIONS, DATASET, DATASET_COLUMN, DATASET_COLUMNS, DATASETS, MANAGED_FOLDER, ML_TASK, PROJECT, SAVED_MODEL, SCENARIO, VISUAL_ANALYSIS.
Other types: CREDENTIAL_REQUEST, PRESET, PRESETS, SEPARATOR.
Note
In the Python recipes, the value of the parameters are the result of JSON deserialization. As such, you’ll only get the following data types: bool, dict, float, int, list, string.
Conditional parameters¶
The visibilityCondition
field allows displaying a parameter based on the value of other parameters. If the condition is
evaluated as True
then the parameter will be displayed. The values of other parameters are accessible via the
model
object, see below.
For example, the following JSON definition of the parameters :
"params": [
{
"name": "sep1",
"label": "Authentication",
"type": "SEPARATOR"
},
{
"name": "useToken",
"label": "Authenticate with token",
"type": "BOOLEAN"
},
{
"name": "username",
"label": "Login",
"type": "STRING",
"visibilityCondition": "!model.useToken"
},
{
"name": "password",
"label": "Password",
"type": "PASSWORD",
"visibilityCondition": "!model.useToken"
},
{
"name": "token",
"label": "Token",
"type": "STRING",
"visibilityCondition": "model.useToken"
},
{
"name": "sep3",
"label": "Reads",
"type": "SEPARATOR"
},
{
"name": "fetchSize",
"label": "Fetch size",
"type": "INT"
}
]
Produces the following form where the fields Token and Login/Password are shown/hidden depending on the state of the Authenticate with token checkbox:
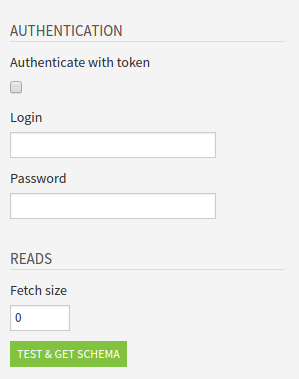
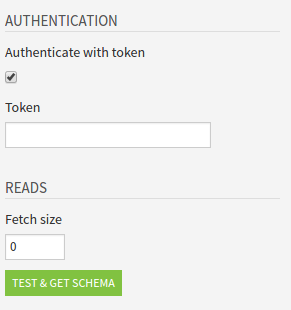
Using visibilityCondition with a SELECT type¶
For example, the following JSON definition of the parameters :
"params": [
{
"name": "prediction_type",
"label": "Regression or Classification",
"type": "SELECT",
"selectChoices" : [
{ "value": "regression", "label": "Regression"},
{ "value": "classification", "label": "Classification"}
],
"mandatory": true
},
{
"name": "target_actual_col",
"label": "Target Column",
"type": "COLUMN",
"columnRole": "input_dataset"
},
{
"name": "prediction_probability_col",
"label": "Prediction Probabilities Column",
"type": "COLUMN",
"columnRole": "input_dataset",
"visibilityCondition": "model.prediction_type == 'classification'"
},
{
"name": "probability_threshold",
"label": "Probability Threshold",
"type": "DOUBLE",
"defaultValue": .5,
"visibilityCondition": "model.prediction_type == 'classification'"
},
{
"name": "prediction_col",
"label": "Predictions Column Name",
"type": "COLUMN",
"columnRole": "input_dataset",
"visibilityCondition": "model.prediction_type == 'regression'"
}
],
produces the following form where the fields Prediction Probabilities Column and Probability Threshold are shown if Classification is selected as the prediction type, and Predictions Column Name is shown if Regression is selected:
Basic types¶
String parameters¶
STRING: A simple string
TEXTAREA: A string, but the UI shows a multi-line control suitable for entering long text.
PASSWORD: A string, but the UI shows a password input field.
STRINGS: A list of strings
PASSWORDS (for example, to access an external API) should typically be plugin parameters, not component parameters, so that they are set only once and visible only to admins. See Plugin-level configuration for details on plugin parameters.
In the case of STRINGS, you may specify:
"allowDuplicates": false
: to prevent duplicate strings in the parameter (case sensitive)
Example:
{
"type": "STRING",
"name": "favorite_quote",
"label": "What's your favorite quote from Camus?",
"defaultValue": "At any street corner the feeling of absurdity can strike any man in the face."
}
Numerical parameters¶
INT: An integer.
DOUBLE: A decimal.
You can provide minI
/maxI
(for INT) and minD
/maxD
(for DOUBLE) to force a value interval.
Example:
{
"type": "INT",
"name": "age",
"label": "Age of the captain",
"minI": 0,
"maxI": 122
}
INTS: A list of integers.
DOUBLES: A list of decimals.
You can not provide minI
/maxI
(for INTS) and minD
/maxD
(for DOUBLES) to force a value interval.
Boolean parameters¶
BOOLEAN: A boolean, displayed as a checkbox.
Example:
{
"type": "BOOLEAN",
"name": "the_other_boolean_g",
"label": "I accept",
"defaultValue": false
}
Date parameters¶
DATE: A simple date, that you will be able to pick from a calendar. This returns a string representing the chosen date, in the Zulu DateTime format.
Example:
{
"type": "DATE",
"name": "release_date",
"label": "Release Date"
}
Complex types¶
Multi-choice parameters¶
SELECT: Select one value among possible choices.
MULTISELECT: Select several values among possible choices.
SELECT and MULTISELECT parameters must have one of the two fields:
selectChoices
: list of{value, label}
if the list is static.getChoicesFromPython
: true if the plugin contains a python function that can generate dynamically the list of{value, label}
. For details see below in the advanced section.
SELECT allows you to offer several choices, and have the user select one (or many, in the case of MULTISELECT).
Each choice has an identifier (value
) and a user-visible long string (label
). It returns a string (or a list of)
representing the value
chosen.
Example of a simple select¶
{
"type": "SELECT",
"name": "egg_type",
"label": "Choose your eggs",
"selectChoices": [
{ "value": "scrambled", "label": "Scrambled"},
{ "value": "sunny_up", "label": "Sunny-side up"}
]
}
Dynamic select using python¶
To populate the choices of a select using a python script, you need to:
specify a SELECT param with
"getChoicesFromPython": true
create a python file under the resource folder of the plugin (ex:
computechoices.py
) and create ado()
function in it that returns a dict with a key “choices”point to the python file from the component JSON file by specifying
"paramsPythonSetup": "computechoices.py"
(replace with the proper filename)
Example:
webapp.json
:... "paramsPythonSetup": "compute_available_time_slots.py" "params": [ { "type": "SELECT", "name": "time_slot", "getChoicesFromPython": true } ], ...
resource/compute_available_time_slots.py
def do(payload, config, plugin_config, inputs): choices = [ { "value": "val1", "label": "Value 1"}, { "value": "val2", "label": "Value 2"} ] return {"choices": choices}
Optional fields¶
By default, the dynamic select will be triggered each time a parameter changes in the form. You may want to restrict the dynamic select trigger behavior using the following options:
disableAutoReload
: The dynamic select python code will only be triggered at the load of the auto-config form and not each time a parameter changes. Use this option as follow:... "paramsPythonSetup": "compute_available_time_slots.py", "params": [ { "name": "param_A", "label": "The param A", "type": "DOUBLE", "defaultValue": .5 }, { "name": "param_B", "label": "The param B", "type": "DOUBLE", "defaultValue": .5 }, { "type": "SELECT", "name": "param_C", "getChoicesFromPython": true, "disableAutoReload": true } ], ...
In the above example, the param_C will only be updated when the form is loaded. Further changes on
param_A
orparam_B
won’t call the python script and thereforefield_C
will stay unchanged.triggerParameters
: Restrict the dynamic select trigger on specific parameter changes. Particularly useful if your dynamic selection only depends on specific parameters. Use this option as follow:... "paramsPythonSetup": "compute_available_time_slots.py", "params": [ { "name": "param_A", "label": "The param A", "type": "DOUBLE", "defaultValue": .5 }, { "name": "param_B", "label": "The param B", "type": "DOUBLE", "defaultValue": .5 }, { "type": "SELECT", "name": "param_C", "getChoicesFromPython": true, "triggerParameters": ["param_B"] } ], ...
In the above example, the
param_C
will only be updated when theparam_B
changes value.
Structured parameters¶
MAP: A (key -> value) mapping. This will return a
dict
of the form :{ 'key1' : 'value1', 'key2': 'value2'}
.KEY_VALUE_LIST: A list of (key -> value). Similar to MAP but with an order. This will return a
list
of the[{'from': 'key1', 'to': 'value1'}, {'from': 'key2', 'to': 'value2'}]
.ARRAY: Let the user choose its input types, and fill an array with these inputs.
OBJECT_LIST: a list of complex objects tied together, it requires an additional field:
subParams
. This will return alist
ofdict
.{ "name": "identity", "label": "Identities", "type": "OBJECT_LIST", "subParams": [ { "type": "SEPARATOR", "label": "Enter your identities" }, { "name": "document_type", "type": "SELECT", "label": "Document type", "selectChoices": [ { "value": "id", "label": "Id Card"}, { "value": "passport", "label": "Passport"} ] }, { "name": "number", "type": "STRING", "label": "Number of your Id card", "visibilityCondition": "model.document_type == 'id'" }, { "name": "number", "type": "STRING", "label": "Number of your Passport", "visibilityCondition": "model.document_type == 'passport'" } ] }
DSS object parameters¶
API_SERVICE: A DSS API service.
API_SERVICE_VERSION: A version package among a specified API service. This type requires an
apiServiceParamName
to point to another parameter that has the type API_SERVICE. See Usage of apiServiceParamName.BUNDLE: An automation bundle.
CLUSTER: A DSS cluster.
CODE_ENV: A DSS code environment.
CONNECTIONS: One or more connections.
DATASET: Select exactly one dataset.
DATASET_COLUMN: A column from a specified dataset. This type requires a
datasetParamName
to point to another parameter that has the type. See Usage of datasetParamName. (Note that this is probably not what you want for recipes, see the COLUMN type).DATASET_COLUMNS: One or more columns from a specified dataset.
DATASETS: One or more datasets.
MANAGED_FOLDER: A DSS managed folder (appears in the flow).
PLUGIN: A DSS plugin.
PROJECT: A Dataiku DSS project
SAVED_MODEL: A DSS saved model (deployed version of a model that appears on the flow)
SCENARIO: A DSS scenario.
VISUAL_ANALYSIS: A visual analysis.
Specific fields:
datasetParamName
: For DATASET_COLUMN(S) only. Parameter name of the related dataset.apiServiceParamName
: For API_SERVICE_VERSION only. Parameter name of the related API Service.canSelectForeign
(boolean, default false): For DATASET(S), SAVED_MODEL and MANAGED_FOLDER only. Should this parameter show elements from other projects?
These objects return either the id or the name to be able to retrieve the information in the associated component code.
Examples¶
Usage of datasetParamName
¶
{
"type": "DATASET",
"name": "mydataset",
"label": "Dataset to analyze"
},
{
"type": "DATASET_COLUMN",
"name": "mycolumn",
"datasetParamName": "mydataset"
"label": "Column in the dataset to analyze"
}
Usage of apiServiceParamName
¶
"params": [
{
"name": "input_dataset",
"type": "DATASET",
"label": "Input dataset"
},
{
"name": "input_api_service",
"type": "API_SERVICE",
"label": "API Service"
},
{
"name": "input_api_service_version",
"type": "API_SERVICE_VERSION",
"apiServiceParamName": "input_api_service",
"label": "API Service version package",
"description": "retrieved from the API Service stated above"
}
]
Selecting columns in plugin recipes¶
In recipes, it’s common to want to select one or several columns from one of the input datasets. This is done using parameter types:
COLUMN: Select exactly one column
COLUMNS: select one or more columns
You will need to give the name of the role from which you want to select a column using columnRole
field. Note that if the given role is multi-dataset, only the columns from the first dataset will be displayed.
Example:
{
"name": "incol",
"label": "Input column",
"type": "COLUMN",
"columnRole": "input_role_1"
}
Optionally, you can use the field allowedColumnTypes
to trigger an error message when the user selects a column with an invalid storage type.
For example, the following COLUMN parameter will raise error messages for non-numerical columns:
{
"name": "numerical_column",
"label": "Numerical column",
"type": "COLUMN",
"allowedColumnTypes": [
"tinyint",
"smallint",
"int",
"bigint",
"float",
"double"
],
"columnRole": "input_dataset",
"mandatory": true
}
You can choose the following storage types : string
, date
, geopoint
, geometry
, array
, map
, object
, boolean
, double
, float
, bigint
, int
, smallint
, tinyint
.
Note
Custom preparation processors do not support the field
allowedColumnTypes
.
Other types¶
Preset parameters¶
You can select some pre-defined values with a PRESET parameter.
When you develop or use a plugin, you may need to share some settings across different components. For example, a custom recipe or a custom dataset may have some common parameters like user credentials. In this case, we recommend using presets to store and interact with these shared parameters.
It is possible to input the value of a PRESET :
At the instance level: The admins of the instance input the values of the presets in the settings tab of the plugin.
At the project level: The administrators of the project input the values in the settings of the project. Settings > Plugins presets.
At the component level: If the settings of the preset allow it, the user can also input the values of the preset in the settings of the component.
To use a PRESET type, you must :
Create a Parameter Set in your plugin. If you edit the plugin with the plugin developer tools, go to the main page of the plugin > click on + New component > Parameter set.
Reference the Parameter set when defining the preset using the field :
parameterSetId
: parameter set of which we should see the values listed.
Example
{
"type": "PRESET",
"name": "aws_account",
"label": "Choose which account to use",
"parameterSetId": "aws_accounts"
}
The Amazon comprehend plugin contains preset parameters. See its source code to see how to set up a preset and a parameter set.
Credential requests¶
CREDENTIAL_REQUEST: This generates a request for a per-user credential. Once a preset of the parameter set is instantiated, each user will then be able to add their credential in Profile > Credentials. This parameter type is only accepted in the
params
section of a parameter set.
A credentialRequestSettings
object must be added to define the credential request. This object contains:
type
: The type of credential. Must be one ofSINGLE_FIELD
,BASIC
, orOAUTH2
For OAUTH2
, the following fields must/may be added to the credentialRequestSettings
object:
authorizationEndpoint
: The authorization endpoint of the OAuth2 authorization servertokenEndpoint
: The token endpoint of the OAuth2 authorization serverscope
: [Optional] Should be a space-delimited stringresources
: [Optional] Rarely used. Must be a list of strings
Additionally, for OAUTH2
, once a preset of this parameter set is instantiated, you must enter in the Client ID and Client Secret (if applicable) that is configured for your registered application on the OAuth2 server. See OAuth2 credentials for more information.
These credentials will then be available for use by plugin code (see Read the settings of a plugin). For example, for a BASIC
credential, the username and password will be available in the settings, while for an OAUTH2
credential, a valid OAuth2 access token will be in the settings.
Examples:
{
"type": "CREDENTIAL_REQUEST",
"name": "basic_credentials",
"label": "Basic credentials",
"credentialRequestSettings": {
"type": "BASIC"
}
},
{
"type": "CREDENTIAL_REQUEST",
"name": "oauth_credentials",
"label": "OAuth2 credentials",
"credentialRequestSettings": {
"type": "OAUTH2",
"authorizationEndpoint": "https://authserver.com/oauth2/authorize",
"tokenEndpoint": "https://authserver.com/oauth2/token",
"scope": "scope1 user.scope2"
}
}
Separators¶
Finally, there is a special parameter type called SEPARATOR, used just for display purposes to separate the form into
sections. The description
field can be used to display additional information. In this description, you can use
HTML tags to format the description.
Fully custom UI¶
You can specify fully custom HTML/JavaScript, see Other topics
Plugin-level configuration¶
Just like each dataset and recipe can accept params, so can a plugin. Plugin-level configuration allows you to have a centralized configuration that is shared by all datasets and all recipe instances of this plugin.
Another characteristic of plugin-level config is that it’s only readable and writable by the Administrator. As such, it can be the right place to store API keys, credentials, connection strings, …
Add settings to a plugin¶
To add settings to a plugin, edit the plugin.json file and add a "params"
array in the JSON top-level object. The structure of this params array is similar to the one of datasets and recipes.
Read the settings of a plugin¶
Datasets receive the plugin config (as a Python dict) in the constructor of their connector class. See the documentation of the Connector class or the automatically generated sample for more information.
Python recipes can read the plugin config (as a Python dict) by calling the
dataiku.customrecipe.get_plugin_config()
functionR recipes can read the plugin config by calling the
dataiku::dkuPluginConfig()
function